Machine Learning for Risk Calculations
A Practitioner's View
Wiley Finance Series
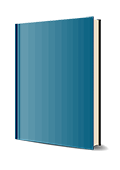
1. Auflage Januar 2022
464 Seiten, Hardcover
Wiley & Sons Ltd
State-of-the-art algorithmic deep learning and tensoring techniques for financial institutions
The computational demand of risk calculations in financial institutions has ballooned and shows no sign of stopping. It is no longer viable to simply add more computing power to deal with this increased demand. The solution? Algorithmic solutions based on deep learning and Chebyshev tensors represent a practical way to reduce costs while simultaneously increasing risk calculation capabilities. Machine Learning for Risk Calculations: A Practitioner's View provides an in-depth review of a number of algorithmic solutions and demonstrates how they can be used to overcome the massive computational burden of risk calculations in financial institutions.
This book will get you started by reviewing fundamental techniques, including deep learning and Chebyshev tensors. You'll then discover algorithmic tools that, in combination with the fundamentals, deliver actual solutions to the real problems financial institutions encounter on a regular basis. Numerical tests and examples demonstrate how these solutions can be applied to practical problems, including XVA and Counterparty Credit Risk, IMM capital, PFE, VaR, FRTB, Dynamic Initial Margin, pricing function calibration, volatility surface parametrisation, portfolio optimisation and others. Finally, you'll uncover the benefits these techniques provide, the practicalities of implementing them, and the software which can be used.
* Review the fundamentals of deep learning and Chebyshev tensors
* Discover pioneering algorithmic techniques that can create new opportunities in complex risk calculation
* Learn how to apply the solutions to a wide range of real-life risk calculations.
* Download sample code used in the book, so you can follow along and experiment with your own calculations
* Realize improved risk management whilst overcoming the burden of limited computational power
Quants, IT professionals, and financial risk managers will benefit from this practitioner-oriented approach to state-of-the-art risk calculation.
Foreword xxi
Motivation and aim of this book xxiii
Part One Fundamental Approximation Methods
Chapter 1 Machine Learning 3
1.1 Introduction to Machine Learning 3
1.1.1 A brief history of Machine Learning Methods 4
1.1.2 Main sub-categories in Machine Learning 5
1.1.3 Applications of interest 7
1.2 The Linear Model 7
1.2.1 General concepts 8
1.2.2 The standard linear model 12
1.3 Training and predicting 15
1.3.1 The frequentist approach 18
1.3.2 The Bayesian approach 21
1.3.3 Testing--in search of consistent accurate predictions 25
1.3.4 Underfitting and overfitting 25
1.3.5 K-fold cross-validation 27
1.4 Model complexity 28
1.4.1 Regularisation 29
1.4.2 Cross-validation for regularisation 31
1.4.3 Hyper-parameter optimisation 33
Chapter 2 Deep Neural Nets 39
2.1 A brief history of Deep Neural Nets 39
2.2 The basic Deep Neural Net model 41
2.2.1 Single neuron 41
2.2.2 Artificial Neural Net 43
2.2.3 Deep Neural Net 46
2.3 Universal Approximation Theorems 48
2.4 Training of Deep Neural Nets 49
2.4.1 Backpropagation 50
2.4.2 Backpropagation example 51
2.4.3 Optimisation of cost function 55
2.4.4 Stochastic gradient descent 57
2.4.5 Extensions of stochastic gradient descent 58
2.5 More sophisticated DNNs 59
2.5.1 Convolution Neural Nets 59
2.5.2 Other famous architectures 63
2.6 Summary of chapter 64
Chapter 3 Chebyshev Tensors 65
3.1 Approximating functions with polynomials 65
3.2 Chebyshev Series 66
3.2.1 Lipschitz continuity and Chebyshev projections 67
3.2.2 Smooth functions and Chebyshev projections 70
3.2.3 Analytic functions and Chebyshev projections 70
3.3 Chebyshev Tensors and interpolants 72
3.3.1 Tensors and polynomial interpolants 72
3.3.2 Misconception over polynomial interpolation 73
3.3.3 Chebyshev points 74
3.3.4 Chebyshev interpolants 76
3.3.5 Aliasing phenomenon 77
3.3.6 Convergence rates of Chebyshev interpolants 77
3.3.7 High-dimensional Chebyshev interpolants 79
3.4 Ex ante error estimation 82
3.5 What makes Chebyshev points unique 85
3.6 Evaluation of Chebyshev interpolants 89
3.6.1 Clenshaw algorithm 90
3.6.2 Barycentric interpolation formula 91
3.6.3 Evaluating high-dimensional tensors 93
3.6.4 Example of numerical stability 94
3.7 Derivative approximation 95
3.7.1 Convergence of Chebyshev derivatives 95
3.7.2 Computation of Chebyshev derivatives 96
3.7.3 Derivatives in high dimensions 97
3.8 Chebyshev Splines 99
3.8.1 Gibbs phenomenon 99
3.8.2 Splines 100
3.8.3 Splines of Chebyshev 101
3.8.4 Chebyshev Splines in high dimensions 101
3.9 Algebraic operations with Chebyshev Tensors 101
3.10 Chebyshev Tensors and Machine Learning 103
3.11 Summary of chapter 104
Part Two The toolkit -- plugging in approximation methods
Chapter 4 Introduction: why is a toolkit needed 107
4.1 The pricing problem 107
4.2 Risk calculation with proxy pricing 109
4.3 The curse of dimensionality 110
4.4 The techniques in the toolkit 112
Chapter 5 Composition techniques 113
5.1 Leveraging from existing parametrisations 114
5.1.1 Risk factor generating models 114
5.1.2 Pricing functions and model risk factors 115
5.1.3 The tool obtained 116
5.2 Creating a parametrisation 117
5.2.1 Principal Component Analysis 117
5.2.2 Autoencoders 119
5.3 Summary of chapter 120
Chapter 6 Tensors in TT format and Tensor Extension Algorithms 123
6.1 Tensors in TT format 123
6.1.1 Motivating example 124
6.1.2 General case 124
6.1.3 Basic operations 126
6.1.4 Evaluation of Chebyshev Tensors in TT format 127
6.2 Tensor Extension Algorithms 129
6.3 Step 1--Optimising over tensors of fixed rank 129
6.3.1 The Fundamental Completion Algorithm 131
6.4 Step 2--Optimising over tensors of varying rank 133
6.4.1 The Rank Adaptive Algorithm 134
6.5 Step 3--Adapting the sampling set 135
6.5.1 The Sample Adaptive Algorithm 136
6.6 Summary of chapter 137
Chapter 7 Sliding Technique 139
7.1 Slide 139
7.2 Slider 140
7.3 Evaluating a slider 141
7.3.1 Relation to Taylor approximation 142
7.4 Summary of chapter 142
Chapter 8 The Jacobian projection technique 143
8.1 Setting the background 144
8.2 What we can recover 145
8.2.1 Intuition behind g and its derivative dg 146
8.2.2 Using the derivative of f 147
8.2.3 When k
8.3 Partial derivatives via projections onto the Jacobian 149
Part Three Hybrid solutions -- approximation methods and the toolkit
Chapter 9 Introduction 155
9.1 The dimensionality problem revisited 155
9.2 Exploiting the Composition Technique 156
Chapter 10 The Toolkit and Deep Neural Nets 159
10.1 Building on P using the image of g 159
10.2 Building on f 160
Chapter 11 The Toolkit and Chebyshev Tensors 161
11.1 Full Chebyshev Tensor 161
11.2 TT-format Chebyshev Tensor 162
11.3 Chebyshev Slider 162
11.4 A final note 163
Chapter 12 Hybrid Deep Neural Nets and Chebyshev Tensors Frameworks 165
12.1 The fundamental idea 165
12.1.1 Factorable Functions 167
12.2 DNN+CT with Static Training Set 168
12.3 DNN+CT with Dynamic Training Set 171
12.4 Numerical Tests 172
12.4.1 Cost Function Minimisation 172
12.4.2 Maximum Error 174
12.5 Enhanced DNN+CT architectures and further research 174
Part Four Applications
Chapter 13 The aim 179
13.1 Suitability of the approximation methods 179
13.2 Understanding the variables at play 181
Chapter 14 When to use Chebyshev Tensors and when to use Deep Neural Nets 185
14.1 Speed and convergence 185
14.1.1 Speed of evaluation 186
14.1.2 Convergence 186
14.1.3 Convergence Rate in Real-Life Contexts 187
14.2 The question of dimension 190
14.2.1 Taking into account the application 192
14.3 Partial derivatives and ex ante error estimation 195
14.4 Summary of chapter 197
Chapter 15 Counterparty credit risk 199
15.1 Monte Carlo simulations for CCR 200
15.1.1 Scenario diffusion 200
15.1.2 Pricing step--computational bottleneck 200
15.2 Solution 201
15.2.1 Popular solutions 201
15.2.2 The hybrid solution 202
15.2.3 Variables at play 203
15.2.4 Optimal setup 207
15.2.5 Possible proxies 207
15.2.6 Portfolio calculations 209
15.2.7 If the model space is not available 209
15.3 Tests 211
15.3.1 Trade types, risk factors and proxies 212
15.3.2 Proxy at each time point 213
15.3.3 Proxy for all time points 223
15.3.4 Adding non-risk-driving variables 228
15.3.5 High-dimensional problems 235
15.4 Results Analysis and Conclusions 236
15.5 Summary of chapter 239
Chapter 16 Market Risk 241
16.1 VaR-like calculations 242
16.1.1 Common techniques in the computation of VaR 243
16.2 Enhanced Revaluation Grids 245
16.3 Fundamental Review of the Trading Book 246
16.3.1 Challenges 247
16.3.2 Solution 248
16.3.3 The intuition behind Chebyshev Sliders 252
16.4 Proof of concept 255
16.4.1 Proof of concept specifics 255
16.4.2 Test specifics 257
16.4.3 Results for swap 260
16.4.4 Results for swaptions 10-day liquidity horizon 262
16.4.5 Results for swaptions 60-day liquidity horizon 265
16.4.6 Daily computation and reusability 268
16.4.7 Beyond regulatory minimum calculations 271
16.5 Stability of technique 272
16.6 Results beyond vanilla portfolios--further research 272
16.7 Summary of chapter 273
Chapter 17 Dynamic sensitivities 275
17.1 Simulating sensitivities 276
17.1.1 Scenario diffusion 276
17.1.2 Computing sensitivities 276
17.1.3 Computational cost 276
17.1.4 Methods available 277
17.2 The Solution 278
17.2.1 Hybrid method 279
17.3 An important use of dynamic sensitivities 282
17.4 Numerical tests 283
17.4.1 FX Swap 283
17.4.2 European Spread Option 284
17.5 Discussion of results 291
17.6 Alternative methods 293
17.7 Summary of chapter 294
Chapter 18 Pricing model calibration 295
18.1 Introduction 295
18.1.1 Examples of pricing models 297
18.2 Solution 298
18.2.1 Variables at play 299
18.2.2 Possible proxies 299
18.2.3 Domain of approximation 300
18.3 Test description 301
18.3.1 Test setup 301
18.4 Results with Chebyshev Tensors 304
18.4.1 Rough Bergomi model with constant forward variance 304
18.4.2 Rough Bergomi model with piece-wise constant forward variance 307
18.5 Results with Deep Neural Nets 309
18.6 Comparison of results via CT and DNN 310
18.7 Summary of chapter 311
Chapter 19 Approximation of the implied volatility function 313
19.1 The computation of implied volatility 314
19.1.1 Available methods 315
19.2 Solution 316
19.2.1 Reducing the dimension of the problem 317
19.2.2 Two-dimensional CTs 318
19.2.3 Domain of approximation 321
19.2.4 Splitting the domain 323
19.2.5 Scaling the time-scaled implied volatility 325
19.2.6 Implementation 328
19.3 Results 330
19.3.1 Parameters used for CTs 330
19.3.2 Comparisons to other methods 331
19.4 Summary of chapter 334
Chapter 20 Optimisation Problems 335
20.1 Balance sheet optimisation 335
20.2 Minimisation of margin funding cost 339
20.3 Generalisation--currently "impossible" calculations 345
20.4 Summary of chapter 346
Chapter 21 Pricing Cloning 347
21.1 Pricing function cloning 347
21.1.1 Other benefits 352
21.1.2 Software vendors 352
21.2 Summary of chapter 353
Chapter 22 XVA sensitivities 355
22.1 Finite differences and proxy pricers 355
22.1.1 Multiple proxies 356
22.1.2 Single proxy 357
22.2 Proxy pricers and AAD 358
Chapter 23 Sensitivities of exotic derivatives 359
23.1 Benchmark sensitivities computation 360
23.2 Sensitivities via Chebyshev Tensors 361
Chapter 24 Software libraries relevant to the book 365
24.1 Relevant software libraries 365
24.2 The MoCaX Suite 366
24.2.1 MoCaX Library 366
24.2.2 MoCaXExtend Library 377
Appendices
Appendix A Families of Orthogonal Polynomials 385
Appendix B Exponential Convergence of Chebyshev Tensors 387
Appendix C Chebyshev Splines on Functions with No Singularity Points 391
Appendix D Computational savings details for CCR 395
D.1 Barrier option 395
D.2 Cross-currency swap 395
D.3 Bermudan Swaption 397
D.3.1 Using full Chebyshev Tensors 397
D.3.2 Using Chebyshev Tensors in TT format 397
D.3.3 Using Deep Neural Nets 399
D.4 American option 399
D.4.1 Using Chebyshev Tensors in TT format 400
D.4.2 Using Deep Neural Nets 401
Appendix E Computational savings details for dynamic sensitivities 403
E.1 FX Swap 403
E.2 European Spread Option 404
Appendix F Dynamic sensitivities on the market space 407
F.1 The parametrisation 408
F.2 Numerical tests 410
F.3 Future work . . . when k > 1 412
Appendix G Dynamic sensitivities and IM via Jacobian Projection technique 415
Appendix H MVA optimisation -- further computational enhancement 419
Bibliography 421
Index 425
MARIANO ZERON, PhD, is Head of Research and Development at MoCaX Intelligence. Prior to that he was a quant researcher at Areski Capital. He has extensive experience with Chebyshev Tensors and Deep Neural Nets applied to risk calculations. He holds a PhD in Mathematics from the University of Cambridge.