Applied Logistic Regression
Wiley Series in Probability and Statistics
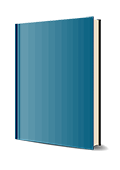
3. Auflage April 2013
528 Seiten, Hardcover
Wiley & Sons Ltd
Kurzbeschreibung
This new edition provides a focused introduction to the LR model and its use in methods for modeling the relationship between a dichotomous outcome variable and a set of covariables. It presents expanded coverage on random effects models, estimation in the presence of interaction, and fractional polynomials; offers discussions on Bayesian logistic regression, likelihood based confidence interval estimates, tests for non-nested models, and multivariable fractional polynomials; includes R language and updated SAS, STATA, and BUGS computer code for analyzing data sets; and more.
A new edition of the definitive guide to logistic regression modeling for health science and other applications
This thoroughly expanded Third Edition provides an easily accessible introduction to the logistic regression (LR) model and highlights the power of this model by examining the relationship between a dichotomous outcome and a set of covariables.
Applied Logistic Regression, Third Edition emphasizes applications in the health sciences and handpicks topics that best suit the use of modern statistical software. The book provides readers with state-of-the-art techniques for building, interpreting, and assessing the performance of LR models. New and updated features include:
* A chapter on the analysis of correlated outcome data
* A wealth of additional material for topics ranging from Bayesian methods to assessing model fit
* Rich data sets from real-world studies that demonstrate each method under discussion
* Detailed examples and interpretation of the presented results as well as exercises throughout
Applied Logistic Regression, Third Edition is a must-have guide for professionals and researchers who need to model nominal or ordinal scaled outcome variables in public health, medicine, and the social sciences as well as a wide range of other fields and disciplines.
1 Introduction to the Logistic Regression Model 1
1.1Introduction, 1
1.2 Fitting the Logistic Regression Model, 8
1.3 Testing for the Significance of the Coefficients, 10
1.4 Confidence Interval Estimation, 15
1.5 Other Estimation Methods, 20
1.6 Data Sets Used in Examples and Exercises, 22
1.6.1 The ICU Study, 22
1.6.2 The Low Birth Weight Study, 24
1.6.3 The Global Longitudinal Study of Osteoporosis in Women, 24
1.6.4 The Adolescent Placement Study, 26
1.6.5 The Burn Injury Study, 27
1.6.6 The Myopia Study, 29
1.6.7 The NHANES Study, 31
1.6.8 The Polypharmacy Study, 31
Exercises, 32
2 The Multiple Logistic Regression Model 35
2.1 Introduction, 35
2.2 The Multiple Logistic Regression Model, 35
2.3 Fitting the Multiple Logistic Regression Model, 37
2.4 Testing for the Significance of the Model, 39
2.5 Confidence Interval Estimation, 42
2.6 Other Estimation Methods, 45
Exercises, 46
3 Interpretation of the Fitted Logistic Regression Model 49
3.1 Introduction, 49
3.2 Dichotomous Independent Variable, 50
3.3 Polychotomous Independent Variable, 56
3.4 Continuous Independent Variable, 62
3.5 Multivariable Models, 64
3.6 Presentation and Interpretation of the Fitted Values, 77
3.7 A Comparison of Logistic Regression and Stratified Analysis for 2 × 2 Tables, 82
Exercises, 87
4 Model-Building Strategies and Methods for Logistic Regression 89
4.1 Introduction, 89
4.2 Purposeful Selection of Covariates, 89
4.2.1 Methods to Examine the Scale of a Continuous Covariate in the Logit, 94
4.2.2 Examples of Purposeful Selection, 107
4.3 Other Methods for Selecting Covariates, 124
4.3.1 Stepwise Selection of Covariates, 125
4.3.2 Best Subsets Logistic Regression, 133
4.3.3 Selecting Covariates and Checking their Scale Using Multivariable Fractional Polynomials, 139
4.4 Numerical Problems, 145
Exercises, 150
5 Assessing the Fit of the Model 153
5.1 Introduction, 153
5.2 Summary Measures of Goodness of Fit, 154
5.2.1 Pearson Chi-Square Statistic, Deviance, and Sum-of-Squares, 155
5.2.2 The Hosmer-Lemeshow Tests, 157
5.2.3 Classification Tables, 169
5.2.4 Area Under the Receiver Operating Characteristic Curve, 173
5.2.5 Other Summary Measures, 182
5.3 Logistic Regression Diagnostics, 186
5.4 Assessment of Fit via External Validation, 202
5.5 Interpretation and Presentation of the Results from a Fitted Logistic Regression Model, 212
Exercises, 223
6 Application of Logistic Regression with Different Sampling Models 227
6.1 Introduction, 227
6.2 Cohort Studies, 227
6.3 Case-Control Studies, 229
6.4 Fitting Logistic Regression Models to Data from Complex Sample Surveys, 233
Exercises, 242
7 Logistic Regression for Matched Case-Control Studies 243
7.1 Introduction, 243
7.2 Methods For Assessment of Fit in a 1-M Matched Study, 248
7.3 An Example Using the Logistic Regression Model in a 1-1 Matched Study, 251
7.4 An Example Using the Logistic Regression Model in a 1-M Matched Study, 260
Exercises, 267
8 Logistic Regression Models for Multinomial and Ordinal Outcomes 269
8.1 The Multinomial Logistic Regression Model, 269
8.1.1 Introduction to the Model and Estimation of Model Parameters, 269
8.1.2 Interpreting and Assessing the Significance of the Estimated Coefficients, 272
8.1.3 Model-Building Strategies for Multinomial Logistic Regression, 278
8.1.4 Assessment of Fit and Diagnostic Statistics for the Multinomial Logistic Regression Model, 283
8.2 Ordinal Logistic Regression Models, 289
8.2.1 Introduction to the Models, Methods for Fitting, and Interpretation of Model Parameters, 289
8.2.2 Model Building Strategies for Ordinal Logistic Regression Models, 305
Exercises, 310
9 Logistic Regression Models for the Analysis of Correlated Data 313
9.1 Introduction, 313
9.2 Logistic Regression Models for the Analysis of Correlated Data, 315
9.3 Estimation Methods for Correlated Data Logistic Regression Models, 318
9.4 Interpretation of Coefficients from Logistic Regression Models for the Analysis of Correlated Data, 323
9.4.1 Population Average Model, 324
9.4.2 Cluster-Specific Model, 326
9.4.3 Alternative Estimation Methods for the Cluster-Specific Model, 333
9.4.4 Comparison of Population Average and Cluster-Specific Model, 334
9.5 An Example of Logistic Regression Modeling with Correlated Data, 337
9.5.1 Choice of Model for Correlated Data Analysis, 338
9.5.2 Population Average Model, 339
9.5.3 Cluster-Specific Model, 344
9.5.4 Additional Points to Consider when Fitting Logistic Regression Models to Correlated Data, 351
9.6 Assessment of Model Fit, 354
9.6.1 Assessment of Population Average Model Fit, 354
9.6.2 Assessment of Cluster-Specific Model Fit, 365
9.6.3 Conclusions, 374
Exercises, 375
10 Special Topics 377
10.1 Introduction, 377
10.2 Application of Propensity Score Methods in Logistic Regression Modeling, 377
10.3 Exact Methods for Logistic Regression Models, 387
10.4 Missing Data, 395
10.5 Sample Size Issues when Fitting Logistic Regression Models, 401
10.6 Bayesian Methods for Logistic Regression, 408
10.6.1 The Bayesian Logistic Regression Model, 410
10.6.2 MCMC Simulation, 411
10.6.3 An Example of a Bayesian Analysis and Its Interpretation, 419
10.7 Other Link Functions for Binary Regression Models, 434
10.8 Mediation, 441
10.8.1 Distinguishing Mediators from Confounders, 441
10.8.2 Implications for the Interpretation of an Adjusted Logistic Regression Coefficient, 443
10.8.3 Why Adjust for a Mediator? 444
10.8.4 Using Logistic Regression to Assess Mediation: Assumptions, 445
10.9 More About Statistical Interaction, 448
10.9.1 Additive versus Multiplicative Scale-Risk Difference versus Odds Ratios, 448
10.9.2 Estimating and Testing Additive Interaction, 451
Exercises, 456
References 459
Index 479
STANLEY LEMESHOW, PhD, is Professor of Biostatistics and Founding Dean of the College of Public Health at The Ohio State University, Columbus, Ohio.
RODNEY X. STURDIVANT, PhD, is Associate Professor and Founding Director of the Center for Data Analysis and Statistics at the United States Military Academy at West Point, New York.