Multiple Imputation and its Application
Statistics in Practice (Band Nr. 1)
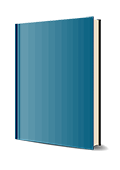
2. Auflage August 2023
464 Seiten, Hardcover
Praktikerbuch
Multiple Imputation and its Application
The most up-to-date edition of a bestselling guide to analyzing partially observed data
In this comprehensively revised Second Edition of Multiple Imputation and its Application, a team of distinguished statisticians delivers an overview of the issues raised by missing data, the rationale for multiple imputation as a solution, and the practicalities of applying it in a multitude of settings.
With an accessible and carefully structured presentation aimed at quantitative researchers, Multiple Imputation and its Application is illustrated with a range of examples and offers key mathematical details. The book includes a wide range of theoretical and computer-based exercises, tested in the classroom, which are especially useful for users of R or Stata. Readers will find:
* A comprehensive overview of one of the most effective and popular methodologies for dealing with incomplete data sets
* Careful discussion of key concepts
* A range of examples illustrating the key ideas
* Practical advice on using multiple imputation
* Exercises and examples designed for use in the classroom and/or private study
Written for applied researchers looking to use multiple imputation with confidence, and for methods researchers seeking an accessible overview of the topic, Multiple Imputation and its Application will also earn a place in the libraries of graduate students undertaking quantitative analyses.
Data acknowledgements xv
Acknowledgements xvii
Glossary xix
Part I Foundations 1
1 Introduction 3
1.1 Reasons for missing data 5
1.2 Examples 6
1.3 Patterns of missing data 7
1.4 Inferential framework and notation 10
1.5 Using observed data to inform assumptions about the missingness mechanism 21
1.6 Implications of missing data mechanisms for regression analyses 24
1.7 Summary 34
2 The Multiple Imputation Procedure and Its Justification 39
2.1 Introduction 39
2.2 Intuitive outline of the MI procedure 40
2.3 The generic MI procedure 45
2.4 Bayesian justification of mi 48
2.5 Frequentist inference 50
2.6 Choosing the number of imputations 55
2.7 Some simple examples 56
2.8 mi in more general settings 64
2.9 Constructing congenial imputation models 72
2.10 Discussion 73
Part II Multiple Imputation for Simple Data Structures 79
3 Multiple Imputation of Quantitative Data 81
3.1 Regression imputation with a monotone missingness pattern 81
3.2 Joint modelling 85
3.3 Full conditional specification 90
3.4 Full conditional specification versus joint modelling 92
3.5 Software for multivariate normal imputation 93
3.6 Discussion 93
4 Multiple Imputation of Binary and Ordinal Data 96
4.1 Sequential imputation with monotone missingness pattern 96
4.2 Joint modelling with the multivariate normal distribution 98
4.3 Modelling binary data using latent normal variables 100
4.4 General location model 108
4.5 Full conditional specification 108
4.6 Issues with over-fitting 110
4.7 Pros and cons of the various approaches 114
4.8 Software 116
4.9 Discussion 116
5 Imputation of Unordered Categorical Data 119
5.1 Monotone missing data 119
5.2 Multivariate normal imputation for categorical data 121
5.3 Maximum indicant model 121
5.4 General location model 125
5.5 FCS with categorical data 128
5.6 Perfect prediction issues with categorical data 130
5.7 Software 130
5.8 Discussion 130
Part III Multiple Imputation in Practice 133
6 Non-linear Relationships, Interactions, and Other Derived Variables 135
6.1 Introduction 135
6.2 No missing data in derived variables 141
6.3 Simple methods 143
6.4 Substantive-model-compatible imputation 152
6.5 Returning to the problems 165
7 Survival Data 175
7.1 Missing covariates in time-to-event data 175
7.2 Imputing censored event times 186
7.3 Non-parametric, or 'hot deck' imputation 188
7.4 Case-cohort designs 191
7.5 Discussion 197
8 Prognostic Models, Missing Data, and Multiple Imputation 200
8.1 Introduction 200
8.2 Motivating example 201
8.3 Missing data at model implementation 201
8.4 Multiple imputation for prognostic modelling 202
8.5 Model building 202
8.6 Model performance 204
8.7 Model validation 206
8.8 Incomplete data at implementation 208
9 Multi-level Multiple Imputation 213
9.1 Multi-level imputation model 213
9.2 MCMC algorithm for imputation model 224
9.3 Extensions 231
9.4 Other imputation methods 234
9.5 Individual participant data meta-analysis 237
9.6 Software 241
9.7 Discussion 241
10 Sensitivity Analysis: MI Unleashed 245
10.1 Review of MNAR modelling 246
10.2 Framing sensitivity analysis: estimands 249
10.3 Pattern mixture modelling with mi 251
10.4 Pattern mixture approach with longitudinal data via mi 263
10.5 Reference based imputation 267
10.6 Approximating a selection model by importance weighting 279
10.7 Discussion 289
11 Multiple Imputation for Measurement Error and Misclassification 294
11.1 Introduction 294
11.2 Multiple imputation with validation data 296
11.3 Multiple imputation with replication data 301
11.4 External information on the measurement process 307
11.5 Discussion 308
12 Multiple Imputation with Weights 312
12.1 Using model-based predictions in strata 313
12.2 Bias in the MI variance estimator 314
12.3 MI with weights 317
12.4 A multi-level approach 320
12.5 Further topics 328
12.6 Discussion 329
13 Multiple Imputation for Causal Inference 333
13.1 Multiple imputation for causal inference in point exposure studies 333
13.2 Multiple imputation and propensity scores 338
13.3 Principal stratification via multiple imputation 343
13.4 Multiple imputation for IV analysis 346
13.5 Discussion 350
14 Using Multiple Imputation in Practice 355
14.1 A general approach 355
14.2 Objections to multiple imputation 359
14.3 Reporting of analyses with incomplete data 363
14.4 Presenting incomplete baseline data 364
14.5 Model diagnostics 365
14.6 How many imputations? 366
14.7 Multiple imputation for each substantive model, project, or dataset? 369
14.8 Large datasets 370
14.9 Multiple imputation and record linkage 375
14.10 Setting random number seeds for multiple imputation analyses 377
14.11 Simulation studies including multiple imputation 377
14.12 Discussion 381
Appendix A Markov Chain Monte Carlo 384
A.1 Metropolis Hastings sampler 385
A.2 Gibbs sampler 386
A.3 Missing data 387
Appendix B Probability Distributions 388
B.1 Posterior for the multivariate normal distribution 391
Appendix C Overview of Multiple Imputation in R, Stata 394
C.1 Basic multiple imputation using R 394
C.2 Basic MI using Stata 395
References 398
Author Index 419
Index of Examples 429
Subject Index 431
JONATHAN W. BARTLETT is a Professor of Medical Statistics at the London School of Hygiene & Tropical Medicine, UK.
TIM P. MORRIS is Principal Research Fellow in Medical Statistics at the MRC Clinical Trials Unit at UCL, UK.
ANGELA M. WOOD is Professor of Health Data Science in the Department of Public Health and Primary Care, University of Cambridge, UK.
MATTEO QUARTAGNO is Senior Research Fellow in Medical Statistics at the MRC Clinical Trials Unit at UCL, UK.
MICHAEL G. KENWARD retired in 2016 after sixteen years as GlaxoSmithKline Professor of Biostatistics at the London School of Hygiene & Tropical Medicine, UK.