Brownian Motion Calculus
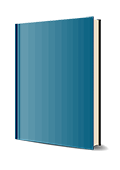
1. Edition April 2008
330 Pages, Softcover
Wiley & Sons Ltd
Short Description
Stochastic (or Brownian motion) calculus provides the formal framework and quantitative tools necessary for modeling stochastic processes (random functions, such as market fluctuations) and has found wide application in financial mathematics for modeling the evolution in time of stock and bond prices. Brownian Motion Calculus provides a short, clear introduction to this subject for readers without a strong mathematical background. Readers with only a basic knowledge of calculus and probability will be able to follow the book's examples and exercises (enhanced with a Web site and disk).
Brownian Motion Calculus
Ubbo Wiersema
Brownian Motion Calculus presents the basics of Stochastic Calculus with a focus on the valuation of financial derivatives. It is intended as an accessible introduction to the technical literature. The sequence of chapters starts with a description of Brownian motion, the random process which serves as the basic driver of the irregular behaviour of financial quantities. That exposition is based on the easily understood discrete random walk. Thereafter the gains from trading in a random environment are formulated in a discrete-time setting. The continuous-time equivalent requires a new concept, the ItM stochastic integral. Its construction is explained step by step, using the so-called norm of a random process (its magnitude), of which a motivated exposition is given in an Annex. The next topic is ItM's formula for evaluating stochastic integrals; it is the random process counter part of the well known Taylor formula for functions in ordinary calculus. Many examples are given. These ingredients are then used to formulate some well established models for the evolution of stock prices and interest rates, so-called stochastic differential equations, together with their solution methods. Once all that is in place, two methodologies for option valuation are presented. One uses the concept of a change of probability and the Girsanov transformation, which is at the core of financial mathematics. As this technique is often perceived as a magic trick, particular care has been taken to make the explanation elementary and to show numerous applications. The final chapter discusses how computations can be made more convenient by a suitable choice of the so-called numeraire. A clear distinction has been made between the mathematics that is convenient for a first introduction, and the more rigorous underpinnings which are best studied from the selected technical references. The inclusion of fully worked out exercises makes the book attractive for self study. Standard probability theory and ordinary calculus are the prerequisites. Summary slides for revision and teaching can be found on the book website www.wiley.com/go/brownianmotioncalculus.
Origins
Notion of a Random Process
Brownian Motion
Stock Price Dynamics
Construction of Brownian Motion from a Discrete Symmetric Random Walk
Features of Brownian Motion Paths
Computations with Brownian motion
References
Examples
2. Martingales
Introduction
Filtration
Conditional Expectation
Martingale
Martingale examples
References
Examples
3. Ito Stochastic Integration
How a Stochastic integral arises in stock trading
Construction of Ito Stochastic integral for random step functions
Extension to general random integrands
Summary of properties of an Ito stochastic integral
Reference
Examples
4. Ito Calculus
Stochastic differential notation
Taylor's expansion in ordinary calculus
Ito's formula as a set of rules
Illustrations of Ito's formula
Justification of Ito's formula
References
Examples
5. Stochastic differential equations
Structure of a stochastic differental equation
Stocastic differntail equations arising in finance
Finding a closed form solution
Checking the solution of an sde
General method for solving sde's
References
Examples
6. Risk-neutral probability
Risk-neutral valuation - the basic concept
Risk-neutral probability construction in discrete one period binomial framework
Risk-neutral probability construction in the continuous framework
Girsanov's theorem
Radon-Nikodym derivative
Numerical Illustration
Motivation for Girsanov's theorem
Summary
References
7. Feynman-Kac Representation
Stochastic Representation
Derivation of simple Feynman-Kac formula
Application to Black Scholes pde
Generalisations
Solution by Simulation
References
Annexes
Computations with Brownian motion
Riemann Integration
Brownian Motion Variability
Norms
Einstrin's Model of Brownian Motion