Geographical Data Imperfection 2
Use Cases
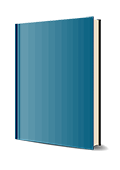
1. Edition March 2024
224 Pages, Hardcover
Wiley & Sons Ltd
Geographical data often contains imperfections associated with insufficient precision, errors or incompleteness. If these imperfections are not identified, taken into account and controlled when using the data, the potential for errors may arise, leading to significant consequences with unforeseeable effects, particularly in a decisionmaking context. It is then necessary to characterize and model this imperfection, and take it into account throughout the process.
In the previous volume, we introduced different approaches for defining, representing and processing imperfections in geographic data. Volume 2 will now present a number of concrete applications in a variety of fields, demonstrating the practical application of the methodology to use cases such as agriculture, natural disaster management, mountain hazards, land management and assistance for the visually impaired.
François PINET, Mireille BATTON-HUBERT and Eric DESJARDIN
Chapter 1 Implementation and Computation of Fuzzy Geographic Objects in Agriculture 1
Mireille BATTON-HUBERT, François PINET and André MIRALLES
1.1 Fuzzy geographic objects 1
1.2 Evaluation of the deposit on crops: formalizing fuzzy data 5
1.3 From the formalization of the problem to the presentation of the objects and their manipulation 6
1.3.1 Materials and methods 7
1.3.2 Elements for the construction of manipulated fuzzy objects and their associated quantities 12
1.4 Implementation and storage of fuzzy objects in a relational database 16
1.5 Some examples of calculations on fuzzy objects 18
1.5.1 Intersection between fuzzy areas 18
1.5.2 Amount of product associated with an alpha-cut of a treatment area 19
1.5.3 Calculating the fuzzy surface of a fuzzy space object 19
1.5.4 Calculation for an alpha-cut of its possible product quantity using its area 20
1.5.5 Going further 22
1.6 Conclusion 23
1.7 References 24
Chapter 2 Representation and Analysis of the Evolution of Agricultural Territories by a Spatio-temporal Graph 25
Aurélie LEBORGNE, Ezriel STEINBERG, Florence LE BER and Stella MARC-ZWECKER
2.1 The data: the land parcel identification system 25
2.2 The model: a fuzzy spatiotemporal graph 27
2.2.1 Graph structure 27
2.2.2 Spatial relationships 28
2.2.3 Spatiotemporal relationships 30
2.2.4 Relationships of filiation 30
2.3 The method: searching for frequent patterns 31
2.3.1 Overview 31
2.3.2 Methods for subgraph mining 32
2.4 Characterizing agricultural regions by spatial-temporal patterns 32
2.4.1 Data from Gers 34
2.4.2 Data from Bas-Rhin 35
2.4.3 Data from Eure-et-Loir 36
2.4.4 Data from the Somme 36
2.5 Conclusion and outlook 38
2.6 References 39
Chapter 3 Agricultural Areas in the Face of Public Environmental Policies: Spatiotemporal Analyses Using Sensitive Data 41
Jean-Michel FOLLIN, Nathalie THOMMERET and Marie FOURNIER
3.1 Project context and issues 42
3.2 What are the methods for anonymization? 44
3.2.1 For the attributes 44
3.2.2 For localization 44
3.3 Data presentation: data in an agricultural context 45
3.4 Treatments at the farm level: spatial structure versus AECM measures 50
3.4.1 Determining the virtual seats 50
3.4.2 Structure analysis: the indicators used 51
3.4.3 Analysis of AECM intensity 53
3.4.4 Introduction of uncertainty at the level of the location by squaring 54
3.5 Treatments at plot level: typology of land changes 57
3.5.1 Construction of a multi-date database 57
3.5.2 Classification 59
3.5.3 Measurement of uncertainty based on class homogeneity percentages 61
3.6 Conclusion and perspectives 64
3.7 Acknowledgments 64
3.8 References 65
Chapter 4 The Representation of Uncertainty Applied to Natural Risk Management 67
Jean-François GIRRES
4.1 Introduction 67
4.2 Natural hazards: uncertain phenomena 68
4.2.1 Risk, hazard and exposure 68
4.2.2 Spatial and temporal uncertainty of natural hazards 70
4.2.3 Sources of uncertainty in natural hazard modeling 71
4.3 Spatial representation of uncertainty: methods and interpretation 73
4.3.1 Representation of uncertain spatial objects 74
4.3.2 Visual variables for representing uncertainty 75
4.3.3 Representation of uncertainty and decision-making 77
4.4 Analysis of uncertainty in natural hazard prevention maps 78
4.4.1 Risk prevention plans 78
4.4.2 Modeling of hazard zones in risk prevention plans 80
4.4.3 Methodology for analyzing the representation of uncertainty 81
4.4.4 Results and comments 82
4.5 Representation of uncertainty in risk maps: assessment and perspectives 86
4.5.1 How uncertain are risk prevention plans? 86
4.5.2 Contributions to the spatial representation of uncertainty 88
4.6 Conclusion 90
4.7 References 91
Chapter 5 Incorporating Uncertainty Into Victim Location Processes in the Mountains: A Methodological, Software and Cognitive Approach 95
Matthieu VIRY, Mattia BUNEL, Marlène VILLANOVA, Ana-Maria OLTEANU-RAIMOND, Cécile DUCHÊNE and Paule-Annick DAVOINE
5.1 Introduction 95
5.2 Sources of imperfection 98
5.2.1 Imprecision in location expression associated with a clue 99
5.2.2 Uncertainty in location expression associated with a clue 100
5.2.3 Incompleteness of geographical data 101
5.3 Detecting uncertainty and imprecision in the interface 101
5.3.1 Formalization of an alert according to the ontologies of the CHOUCAS project 102
5.3.2 Specific acquisition components 104
5.4 Taking imperfection in spatialization into account 107
5.4.1 Relationship between CLZ and PLZ and the construction of the PLZ 107
5.4.2 The process of creating CLZs 108
5.4.3 Taking imprecision into account 109
5.4.4 Taking uncertainty and incompleteness into account 111
5.5 Restoring uncertainty in the interface 112
5.5.1 "Classic" solution 112
5.5.2 Solution based on figures of varying sizes 114
5.5.3 Solution by combining representations 116
5.5.4 Illustration of the Grand Veymont Alert 118
5.6 Conclusion and perspectives 122
5.7 References 123
Chapter 6 Uncertainties Related to Real Estate Price Estimation Scales 127
Didier JOSSELIN, Delphine BLANKE, Mathieu COULON, Guilhem BOULAY, Laure CASANOVA ENAULT, Antoine PERIS, Pierre LE BRUN and Thibault LECOURT
6.1 Introduction 127
6.2 The effect of spatial support in real estate price estimation 129
6.2.1 The generation of uncertainty in the choice of aggregation scales 129
6.2.2 Real estate price representation scales rarely questioned 131
6.2.3 Different scales of real estate price structuring 133
6.3 Data and indicators for estimating the sensitivity of house prices to the scale of aggregation 135
6.3.1 Different territorial grids to test the effects of aggregation 135
6.3.2 A national database of geolocalized real estate transactions in France: DVF 140
6.3.3 Representation of aggregated statistics in the form of scalograms 143
6.4 Methodology for studying variations in real estate price estimates according to scale 144
6.4.1 Preliminary methodological considerations 144
6.4.2 A random sample generator to eliminate scale uncertainty 145
6.4.3 Presentation of analysis elements in the form of a composite graph 147
6.5 Results: highlighting structural effects linked to territorial units and scale salience 148
6.5.1 An analysis of changes in estimates of average and median prices for apartments and houses in the Provence Alpes Côte d'Azur region from 2014 to 2020 148
6.5.2 Analysis of changes in standard deviation estimates of average prices for apartments and houses in the
Provence Alpes Côte d'Azur region from 2014 to 2020 151
6.5.3 Cross-sectional analysis on composite graphs 155
6.6 Conclusion and discussion 157
6.7 References 160
Chapter 7 Representing Urban Space for the Visually Impaired 165
Lisa DENIS, Jérémy KALSRON and Jean-Marie FAVREAU
7.1 Introduction 165
7.2 Landmarks as tools for moving around and finding your location 166
7.2.1 Gathering needs and uses 167
7.2.2 Modeling landmarks and their uses 169
7.2.3 Model expressiveness 175
7.3 Integration of landmarks in tactile and multimodal maps 177
7.3.1 Background map 177
7.3.2 Integration of landmarks 180
7.4 Integrating uncertainty into text descriptions 183
7.4.1 Integrating the probability of detecting landmarks 183
7.4.2 Integrating blurred distances and angles 184
7.5 Conclusion and perspectives 186
7.6 Acknowledgments 187
7.7 References 187
List of Authors 189
Index 193
Mireille Batton-Hubert is a professor at the École Nationale Supérieure des Mines de Saint- Étienne, France.
Eric Desjardin is a lecturer at the University of Reims Champagne-Ardenne within the STIC Research Center (CReSTIC), France.